4 key differences between consumer and enterprise biometrics (Part 1)
By now, we’re all pretty familiar with biometrics as a way to measure a person's physical characteristics to verify their identity. Most of us use biometrics daily to log in to our mobile devices via a fingerprint or face scan or to activate a home personal assistant device like Amazon Alexa or Google Home using our voice. These are popular methods of consumer biometrics.
Some enterprises rely on these methods of consumer biometrics for identification because they are familiar, easy, and commonly stored within the individual’s personal device. However, these types of biometrics may raise security concerns for enterprises, as they are more susceptible to fraud and hacking than enterprise-grade biometrics.
For healthcare, in particular, biometrics are critical to enabling and validating the true digital identity of clinicians and patients, allowing for high-trust, secure and convenient access to patient information and the delivery of care inside and outside the hospital. As a result, healthcare delivery organizations are increasingly relying on methods of enterprise biometrics, such as a palm-vein scan or facial recognition, to ensure both security and convenience.
To help you differentiate between (a) consumer and (b) enterprise biometrics, I’d like to outline 4 key differences between the two classes of biometrics, which are
- Accuracy
- Spoof Detection
- Security & Assurance
- Device Portability and Enterprise Management
In this post, I’ll start by addressing (1) Accuracy and (2) Spoof Detection.
1. Accuracy
To determine how accurate a biometric is, one must look at two key metrics: False Acceptance Rate (FAR) and False Rejection Rate (FRR). Together these two metrics help quantify how well a biometric system performs.
FAR reflects how likely a biometric system is to accept the wrong biometric, a biometric belonging to a user other than the registered user. This speaks to the security aspect of the biometric and its ability to protect a user's account from unauthorized access. You will typically see this written as a ratio, for example 1:10,000 FAR.
The FRR has an impact on usability. It’s the rate at which the correct user’s biometric is rejected. If this is too high, a user will become frustrated and not want to use the biometric. How many times have you yelled at your home technology because it did not detect you properly? This is typically measured in terms of percentage, for example 5% FRR.
It is important to note that FAR and FRR have a direct impact on one another. As an algorithm is tuned for a more stringent FAR (security), the FRR (usability) will increase and vice versa. One difference between a consumer biometric and an enterprise biometric is how the FAR and FRR are biased. A consumer-based biometric algorithm may choose to leverage a more favorable FRR to optimize usability and thus sacrifice a bit of security by setting the algorithm for a higher FAR. In contrast, an enterprise biometric will tend to err more on the security side and sacrifice a bit of UX. In the end, this will vary depending on the use case and the nature of the data being protected by the biometric. The more important the data, the higher the security bar becomes.
When you see vendors talk about their algorithms, you will see them denote performance as, for example, 1% FRR @ FAR 1:10,000.
You will see that for enterprises, they will target a 3% FRR @ FAR 1:100,000 or better. For example, the Palm vein-based biometric from Imprivata is 1% FRR @ FAR 1:1.25 Million.
2. Anti-Spoof Detection
A very key component that separates consumer and enterprise-grade biometrics from one another is anti-spoofing. Anti-spoofing is different from biometric accuracy in that it focuses on attacks from fraudsters that are presenting a high-quality copy of your biometric. Yes, your biometric potentially could be copied like in the movies!
These attacks can range from primitive to extremely sophisticated. The attacks and countermeasures will vary depending on the biometrics. I will focus on face for a moment since that’s something that is readily available just by grabbing someone's LinkedIn profile picture.
The most basic attack involves presenting a picture of someone's face to a phone or PC and hoping the system will think it is the user and allow the fraudster in. To protect against a lot of common attacks like this, companies leverage hardware such as IR cameras that can easily detect that a printed piece of paper or phone with the user's image is being presented. But what if the attacker is very persistent? Well, that’s where enterprise anti-spoofing comes into play.
At the enterprise level, attacks are caught in software using advanced AI algorithms that are either active or passive. Active algorithms ask the user to perform actions such as a blink, a smile, or a turn of the head. Passive algorithms check for natural face motions such as blinking and micro movements in the face as well as reflections, changes in skin tones, facial structure, and much more, without you even knowing it. These algorithms are so advanced that they can detect spoof attempted by imposters wearing life-like (and expensive!) masks such as the ones shown below. When it comes to protecting enterprise data, anti-spoofing is a vital component.
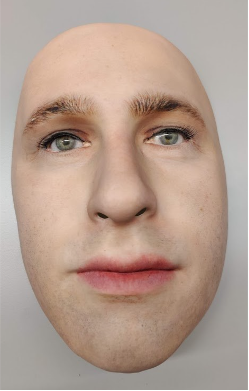
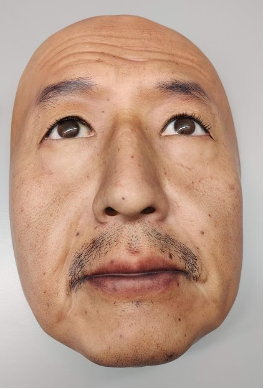
So there you have the first two key differences between consumer and enterprise biometrics. In my next post, I’ll focus on the final three key differences: Security & Assurance, Device Portability, and Enterprise Management. Stay tuned!